Responsable
Fecha inicio
Fecha fin
Grupo
División
One of the objectives intended to be achieved through the development of this subproject is to continue characterizing the in vitro mechanical properties of tissues present in the ankle joint of a mouse model and extend the study to those involved in the shoulder's rotator cuff. In the case of muscles, the active behavior will also be analyzed by inducing contraction through an external electrical signal.
These properties will allow for the quantitative assessment of the degeneration and regeneration process of tissues after surgically inducing a tendon injury and applying different pharmacological treatments. In view of previous results obtained by the research team, to reproduce the mechanical behavior of the tissues through computational, simulation models, it is essential to have accurate reproductions of their anisotropy characterized by their internal structure associated with the presence of collagen fibers. This fact also applies to the mechanical behavior of polymeric scaffolds designed for damage treatment, as a correct formulation of the constitutive law that defines the relationship between the stress state and deformation of the printed fibers is key to determining their resistance capacity. Thus, a significant effort will be needed in the experimental characterization and formulation of models that consider the structural complexity of all analyzed tissues and materials.
In addition to the experimental mechanical characterization of healthy tissue, the aim is to also study the degeneration process by complementing previous work and analyzing new scenarios for the shoulder joint. Measuring this degeneration process in samples from a control group will enable the formulation of a predictive model, based on the finite element method, for muscle tissue damage. Identical strategies will be adapted to analyze the regeneration process, incorporating the polymeric scaffold in both experimental and computational models.
Given the complexity inherent in computational modeling and the associated computational costs, the aim is to create a simulation environment that can make real-time predictions for this type of injury. To achieve this, this subproject aims to explore the potential of machine learning, specifically neural networks, and model order reduction techniques, to develop a clinically applicable tool for predicting suitable personalized treatments and their evolution.
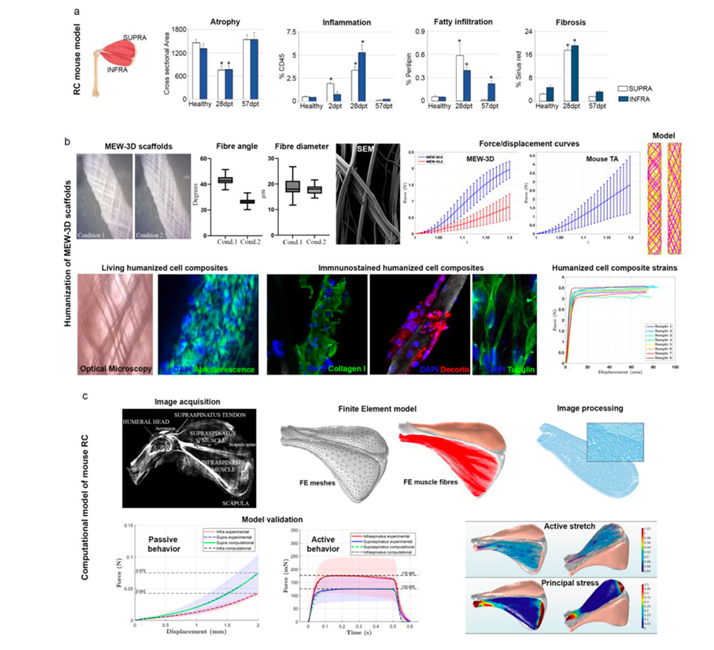
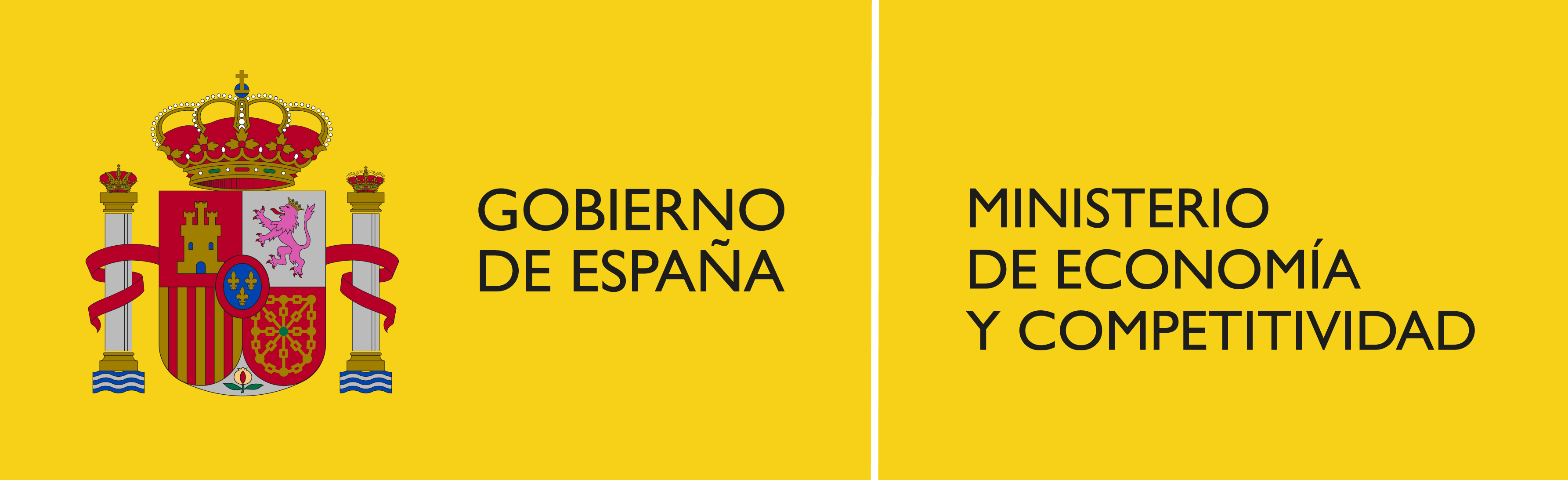