Responsable
Fecha inicio
Fecha fin
Grupo
División
Cardiovascular diseases (CVD) have been the leading cause of death and disability in developed countries for the last decades. In the European Union they represent the main reason for reduced life expectancy. Aneurysm in the descending and thoracic aorta, consisting of a progressive dilatation of the artery, is one of the most serious pathologies associated with a high risk of fatal outcomes such as dissection and rupture of the wall. These events have a very poor prognosis with mortality rates of around 60-70%. All efforts dedicated to increasing knowledge about the appearance and evolution of these pathologies, as well as their diagnosis and application of the most appropriate treatment in each case will have a high impact on the patient and consequently on society as a whole.
The main aim of this project, together with the group of clinical collaborators, is to develop a computational-experimental framework to improve the prediction of the initiation, progression and final outcome of the pathology. The tool will include, on the one hand, parameters already used in the clinic practice such as aortic diameter evolution, growth rate, clinical history and analysis and patient risk factors, with new mechanically-based indicators, such as studies of aortic hemodynamics, analysis of the deformation map, local elastic parameters of the wall and tensional analysis. All this will be done for a patient’s cohort selected by the clinical service that will provide clinical PET/CMR, MRA and 4D flow imaging as well as patient follow-up for several years. The combination of these disciplines will make it possible to determine fundamental aspects in the progression of the disease that may lead to finding more reliable biomarkers in the prognosis of the pathology. The ultimate goal of the project is to apply deep learning techniques to this knowledge in order to incorporate it into a digital twin model. This model can be progressively improved as more data is obtained, whether clinical, experimental or based on computational simulation techniques.
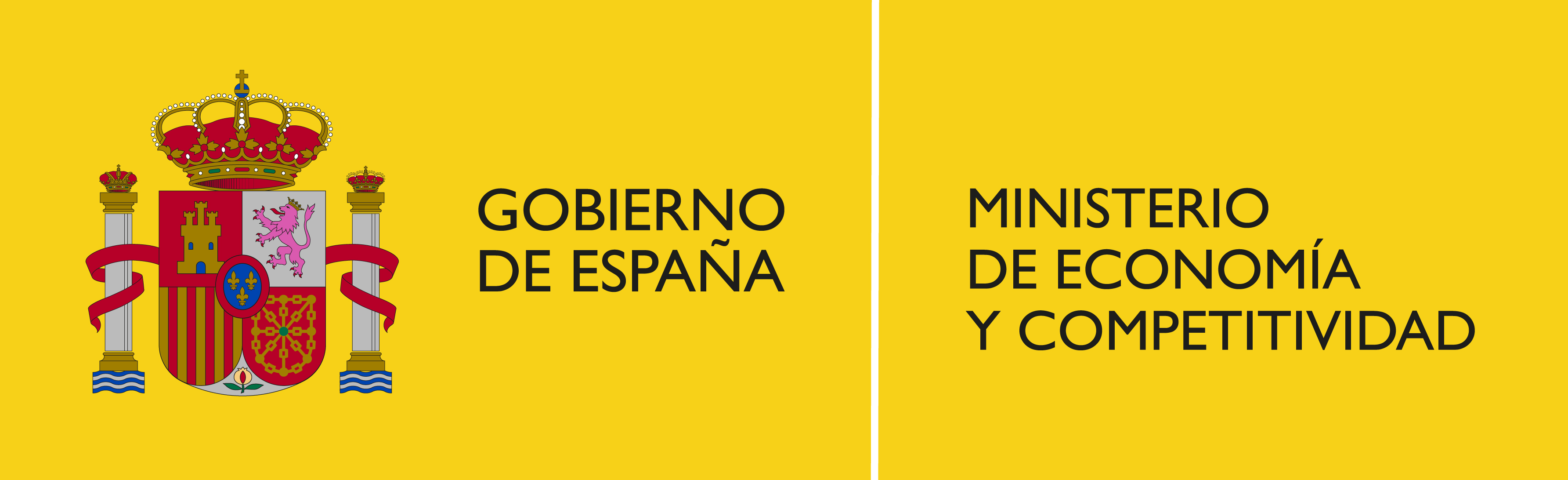